Why RAG Won't Solve Generative AI's Hallucination Problem
RAG is not a complete solution for generative AI hallucinations due to inherent technical limitations.
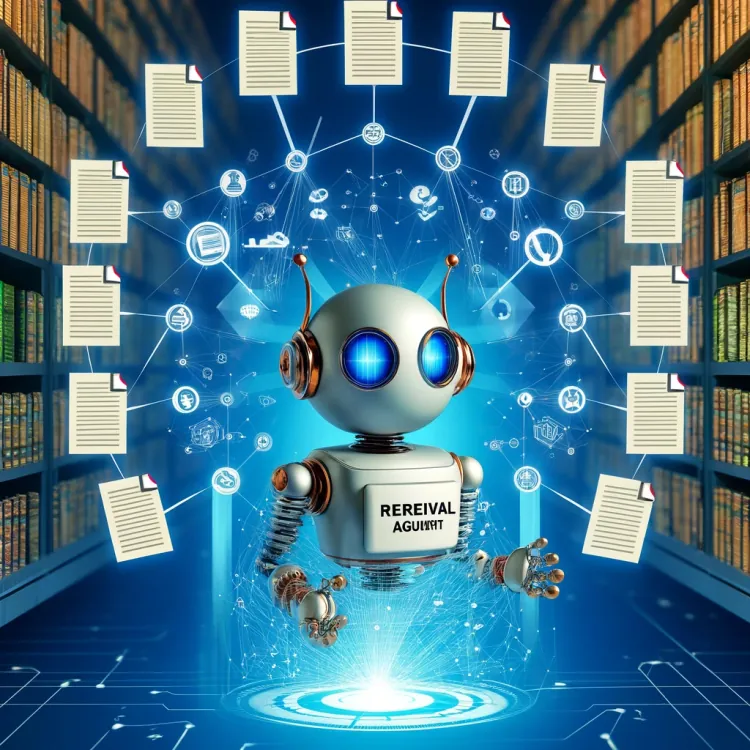
Table of Contents
- I. Introduction
- II. The Concept of Hallucination in AI
- III. What is RAG?
- IV. Limitations of RAG
- V. Technical and Practical Challenges
- VI. Efforts to Improve RAG
- VII. Conclusion
- VIII. FAQs
I. Introduction
Generative AI technologies have increasingly become integral to business operations, yet they are plagued by a significant issue: hallucinations—misinformation generated by AI systems. While Retrieval Augmented Generation (RAG) has been pitched as a remedy, it has distinct limitations.
II. The Concept of Hallucination in AI
AI hallucinations refer to inaccuracies or fabrications in the content produced by AI models, such as generating nonexistent meeting attendees or misreporting discussion topics. These errors can severely impact the credibility and operational efficiency of businesses using AI.
III. What is RAG?
Retrieval Augmented Generation (RAG) is a technology that enhances generative AI by pulling in relevant documents to inform its responses, ostensibly reducing errors by grounding responses in sourced material.
IV. Limitations of RAG
While useful in straightforward, knowledge-based queries (like historical facts), RAG struggles with more complex tasks requiring reasoning or abstract thinking. The technology’s dependency on keyword-matching can miss nuanced or conceptually complex inquiries.
V. Technical and Practical Challenges
Applying RAG broadly is resource-intensive, requiring substantial computational power and hardware for document retrieval and processing. Additionally, the AI might ignore helpful documents or extract incorrect information from them, still leading to potential errors due to reliance on parametric memory.
VI. Efforts to Improve RAG
Researchers are exploring methods to enhance RAG’s efficiency and accuracy, including better indexing of documents and improving AI's decision-making about when and how to use retrieved information. However, these improvements are still in development.
VII. Conclusion
RAG represents a significant advancement in addressing generative AI’s reliability issues, yet it is not a panacea. The complexity of AI hallucinations requires a multifaceted approach beyond current capabilities.
VIII. FAQs
- Q: What is generative AI?
- A: Generative AI refers to artificial intelligence systems that can generate text, images, and other content based on learned data.
- Q: How does RAG work?
- A: RAG works by retrieving external documents related to a query to provide a factual basis for the AI's responses.
- Q: Can RAG completely prevent AI hallucinations?
- A: No, while RAG reduces the frequency of hallucinations by providing sourced content, it does not eliminate the issue entirely, especially in complex scenarios.
What's Your Reaction?
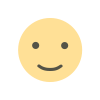
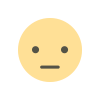
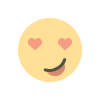
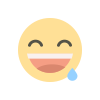
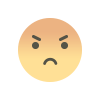
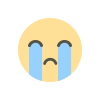
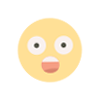